The use of data science in the fields of marketing and e-commerce is widely known for its effectiveness in predicting customer behaviors such as churn rates and preferred offers. However, predictive analytics can also have a significant social impact. A notable example is the partnership between DrivenData, Yelp, and Harvard University, who collaborated to design a solution that could address the issue of health inspections in restaurants in Boston in 2015. The traditional process of conducting health inspections is often time-consuming and randomized checks may not target the establishments at a higher risk of health code violations.
In this case, data scientists gathered information from social media and review sites, such as Yelp, and combined it with historical data of hygiene violations to identify the patterns, words, and phrases that could predict violations. By doing so, public health inspectors were able to focus their efforts and resources more effectively.
This project was particularly important since, according to the Centers for Disease Control, approximately 48 million Americans are affected by foodborne illnesses each year, and an estimated 75% of outbreaks originate from caterers, delis, and restaurants. Predictive analytics is a valuable tool that involves using data to predict future events and outcomes. If you’re interested in mastering some of the top 10 predictive analytics techniques, we encourage you to learn more!
What Is Predictive Analytics?
Predictive analytics is a powerful tool that uses historical data to train machine learning models that can predict future outcomes. This innovative approach is rapidly gaining popularity across all industries as it offers companies a way to solve problems and discover new insights from their data.
Predictive modeling involves approximating a complex mathematical function from input variables to output variables. Common use cases include optimizing marketing campaigns, detecting fraud, forecasting inventory, and setting prices. Airlines, for example, use predictive analytics to set ticket prices based on seat availability and demand.
The global Predictive Analytics market is set to reach $10.95 billion by 2022, indicating that this is a rapidly growing field. Statistics show that businesses are not capturing the full potential of big data for which the demand for both data analysts and data scientists is increasing.
One company that has already made strides in this area is Starbucks, which has become known as a data tech company and uses data to make strategic decisions such as delivering personalized promotions and determining store locations. Predictive analytics also helps businesses minimize risk and detect criminal behavior.
Top 10 Predictive Analytics Techniques
Predictive analytics uses a variety of statistical techniques, as well as data mining, data modeling, machine learning, and artificial intelligence to make predictions about the future based on current and historical data patterns. These predictions are made using machine learning models like classification models, regression models, and neural networks.
1. Data mining
Data mining is a technique that combines statistics and machine learning to discover anomalies, patterns, and correlations in massive datasets. Through this process, businesses can convert raw data into business intelligence—real-time data insights and future predictions that inform decision-making. Data mining involves sifting through repetitive, noisy, unstructured data and discovering patterns that surface relevant insights. Exploratory data analysis (EDA) is a type of data mining technique that involves analyzing datasets to summarize their main characteristics, often with visual methods. EDA does not involve any hypothesis testing or the intentional search for a solution; it is about probing the data in an objective manner, with no expectations. Traditional data mining, on the other hand, focuses on finding solutions from the data or solving a predefined business problem using data insights.
2. Data warehousing
Data warehousing is the foundation of most large-scale data mining efforts. A data warehouse is a type of data management system designed to enable and support business intelligence efforts. It does this by centralizing and consolidating multiple data sources, such as application log files and transactional data from POS (point of sale) systems. Typically, a data warehouse consists of a relational database to store and retrieve data, and an ETL (Extract, Transfer, Load) pipeline to prepare the data for analysis, statistical analysis tools, and client analysis tools for visualizing the data and presenting it to clients.
3. Clustering
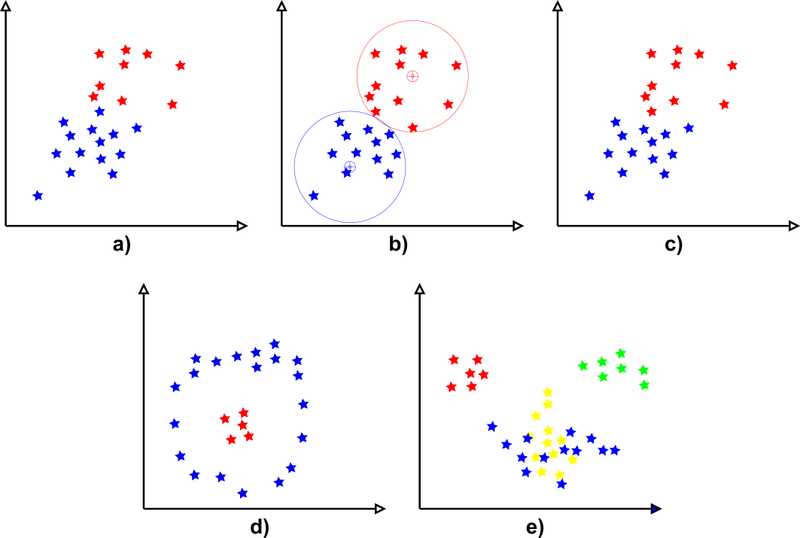
Clustering is one of the most popular data mining techniques, which uses machine learning to group objects into categories based on their similarities, thereby splitting a large dataset into smaller subsets. For example, clustering customers based on similar purchase habits or lifetime value, thereby creating customer segments and enabling the business to create personalized marketing campaigns at scale. Hard clustering means data points are directly assigned to categories. Soft clustering assigns a probability that a data point belongs in one or more clusters, rather than assigning that data point to a cluster. K means clustering is one of the most popular unsupervised machine learning algorithms. This technique involves looking for a fixed number of clusters in a dataset based on a target number ‘k.’ Each data point is allocated to a cluster by reducing the in-cluster sum of squares.
4. Classification
Classification is a fascinating technique that allows us to analyze data and predict the likelihood of a particular item belonging to a specific category. Depending on the nature of the classification problem, there can be two types: binary classification and multi-class classification. Binary classification refers to a problem where there are only two possible classes, whereas multi-class classification deals with more than two classes.
In both cases, classification models are used to generate a continuous value that represents the probability of an observation belonging to a particular category. This value is also known as confidence, and it can be converted into a class label by choosing the class with the highest probability.
Commercially, classification is utilized in various use cases, such as spam filters that classify incoming emails into ‘spam’ or ‘not spam’ categories based on predefined rules, or fraud detection algorithms that single out anomalous transactions. Classification is truly an important technique that has numerous practical applications.
5. Predictive modeling
Predictive modeling is a highly sophisticated, data-driven approach to statistical modeling that has revolutionized the way that businesses approach decision-making. By leveraging cutting-edge analytical tools and techniques, predictive modeling helps organizations anticipate future events and outcomes with remarkable accuracy, providing valuable insights that inform everything from day-to-day operations to long-term strategic planning.
With predictive modeling, businesses can create more effective marketing strategies, optimize supply chains, manage inventory with greater efficiency, and deliver outstanding customer support. Armed with the insights afforded by these powerful predictive models, business leaders can make more informed decisions, improve performance, and achieve exceptional results. So, whether you’re a small start-up or a large multinational corporation, predictive modeling is a must-have tool in your business arsenal that can help you stay ahead of the curve and drive your organization’s success.
6. Logistic regression
Logistic regression modeling is one of the primary tools for predictive modeling. The main purpose of regression techniques is to find correlations between inputs and outputs in the form of a linear expression that describes the strength of the relationship in the form of a mathematical formula. The formula expresses the outputs as a function of the inputs plus a constant.
This linear relationship is then used to predict the future numerical value of a variable. For example, a regression model can show the correlation between house prices and interest rates, and use the linear expression to predict future house prices given interest rate ‘X.’ The variable that is being predicted is called the dependent variable, while the factors used to predict the value of the dependent variable are known as independent variables. There are two types of regression models: simple linear regression (one dependent variable and one independent variable) and multiple linear regression (one dependent variable and multiple independent variables).
7. Decision trees
A decision tree is a supervised learning algorithm and a popular method for visualizing analytical models. Decision trees assign inputs to two or more categories based on a series of “if…then” statements (known as indicators) arranged in the form of a flow diagram. A regression tree is used to predict continuous quantitative data, such as a person’s income. Qualitative predictions involve a classification tree, such as a tree that predicts a diagnosis based on a person’s symptoms. The goal of using a decision tree is to create a training model that can predict the class or value of an input variable by learning simple decision rules inferred from training data.
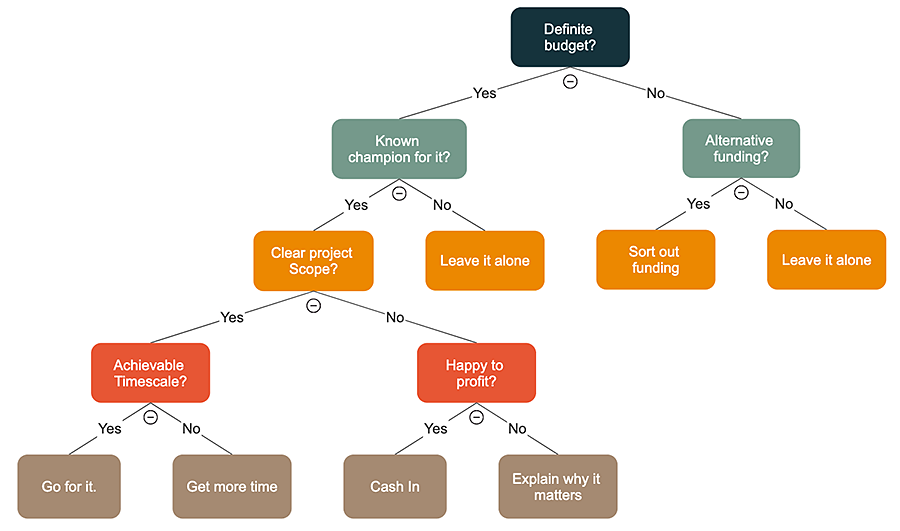
8. Time series analysis
Time series analysis is a method for analyzing time-series data. Time series models predict future values based on previously observed values. A time series is a sequence of data points that occur over a period of time—for example, changes in average household income or the price of a share over time. In this case, the predicted values occur along a continuum relative to time. They are most useful for predicting behavior or metrics over a period of time, or in decisions that involve a factor of uncertainty over time.
9. Neural networks
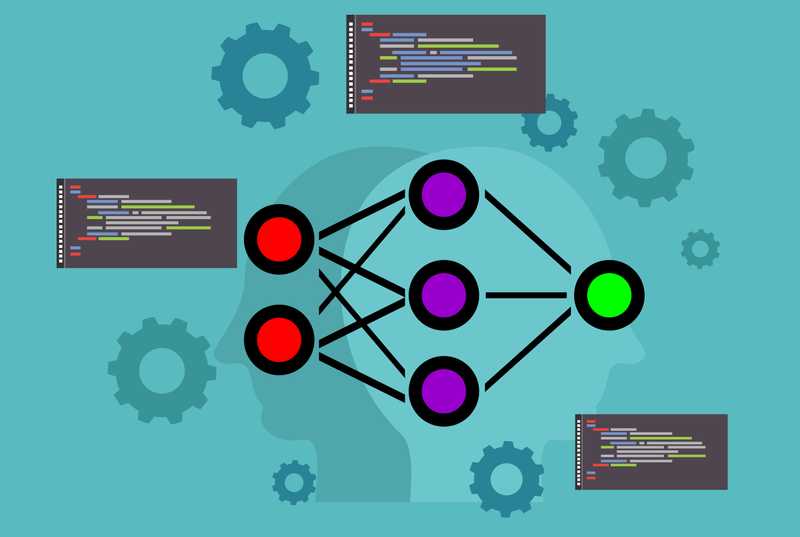
Widely used for data classification problems, neural networks are biologically inspired by the human brain. Most neural networks use mathematical equations to activate the neurons, where each input corresponds to an output. A neural network is made by creating a web of input nodes (which is where you insert the data), output nodes (which show the results when the data has passed through the network), and a hidden layer between these nodes. The hidden layer is what makes the network smarter than traditional predictive tools, because it “learns” the way a human would, by remembering past connections in data and incorporating this data in the algorithm. However, this hidden layer represents a ‘black box,’ meaning that even data scientists cannot necessarily understand how the algorithm produces its computations—only the inputs and outputs can be observed directly.
10. Artificial intelligence and machine learning
One of the key advantages of machine learning is that it can handle large amounts of data, making it ideal for applications such as image recognition, speech analysis, and natural language processing. However, the models generated by machine learning are often difficult to interpret, as they are essentially black boxes. This means that the model is generated directly from the data, without relying on explicit programming by humans.
To ensure the accuracy of machine learning techniques, it is crucial to have high-quality training data. This means data that is not biased, outdated or inadequately represents the target population. If the data is of poor quality, the accuracy of the model’s predictions will be compromised.
Despite these challenges, machine learning remains an invaluable tool for data analysis. By deriving patterns from millions of observations, it can help train the model to recognize patterns in data that it has not yet encountered, making it a powerful tool for predictive modeling.
Benefits of Predictive Analytics
- Personalize the customer experience. Mobile payments, online transactions, and web analytics allow companies to capture troves of data about their customers. In return, customers expect businesses to understand their needs, form relevant interactions, and provide a seamless experience across all touchpoints. Needs forecasting is a popular predictive analytics tool, in which businesses anticipate customer needs based on inferences such as their web browsing habits. For example, retailers like Target are able to predict when a customer is expecting a baby and proactively market baby products to them. Meanwhile, recommendation engines use predictive analytics to guess what types of products you’ll like based on your previous consumption habits or stated preferences.
- Mitigate risk and fraud. Insurance companies and financial institutions use predictive analytics to determine a customer’s “risk” level when evaluating them for an insurance policy or a loan, for example. By aggregating internal customer data and personal data from third-party data brokers—such as an applicant’s credit score, criminal record, and employment history—these businesses use predictive analytics models to determine the likelihood that a loan applicant will default, or the probability that a person of advanced age with certain pre-existing conditions will require intense medical care in the future. Typically, the model generates a risk score, which is then used to determine things like the interest rate on a mortgage, what credit limit to place on a credit card, or how much to charge for a monthly insurance premium. The more “high-risk” an applicant is deemed, the higher their interest rate or premium, thereby helping to insulate the business from risk.
- Proactively address problems. Predictive analytics enables businesses to determine which customers are at risk of churn, which assets are likely to break down, and times of the year when demand will spike (or drop). By forecasting these events in advance, businesses can adopt a proactive rather than reactive approach to addressing problems, thereby minimizing their impact on the customer experience and the bottom line.
- Reduce the time and cost of forecasting business outcomes. Given enough historical data, predictive forecasting can save businesses from engaging in costly, time-consuming activities such as A/B testing, or allocating marketing resources to campaigns that may or may not generate leads. Obviously, the more historical data you feed into a model, the more accurately it can predict future outcomes, but simple linear regression models or decision trees, for example, don’t require datasets collected over a decades-long period in order to generate relatively accurate forecasts.
- Gain a competitive advantage. Businesses that use predictive analytics not only benefit from knowing what happened but why it happened. These are crucial steps to solving business problems and proactively addressing customer needs. Predictive analytics provides proactive insights on what to do next based on forecasts of the future. This can help businesses make better long-term strategic plans while reducing costs and risks. In day-to-day business operations, predictive analytics also helps with efficiency. For example, proper demand forecasting leads to better inventory management, reducing the likelihood of overstocking items or losing sales.